Is your future income written in your DNA?
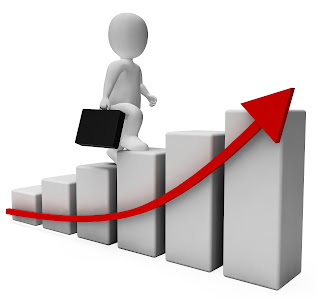
The devil is in the details, of course, of
the methodology and the results, and, importantly, the way they are presented
and interpreted.
The idea that something like a person’s
income could be partly heritable – that is, that variation in income across the
population could be partly attributable to genetic differences between people –
is in fact, not new at all and really not remarkable at this stage. Decades of
research in behavioral genetics has clearly shown that pretty much every human
behavioral trait and every life outcome – from income to marriage to divorce
to educational attainment to going to prison – is partly heritable. This is
because these experiences are partly driven by our psychological traits, which
are themselves partly driven by genetic differences.
Given the existence of these genetic
effects, it may be interesting to try and figure out the underlying mechanisms.
That starts with identifying some of the actual genetic variants that are
associated with the metric of interest in some population, and then figuring
out the causal mechanisms that link them to the phenotype. I say “may be”
interesting because it’s not actually obvious that it always is – sometimes the
details of the genes involved are very informative and others times they’re
really not. Of course, you can’t know until you look.
The paper in question, by W. David Hill and
colleagues, describes the results of a genome-wide association study (GWAS) of
income in a large sample of over 280,000 people from the UK (all ethnically
“white”). GWAS analyse sites in the genome where there is a common variant –
the DNA “letter” at that position may be an “A” in some people and a “C” in
others, for example. The test is simply to see if the frequency of those
variants differs in people with high income versus low income. (In the same way
that the frequency of smoking varies in people with lung cancer versus
without). GWAS look at millions of such sites (known as “SNPs”) across the
genome across the whole sample of hundreds of thousands of people. As a result
of the huge sample, these studies can detect even small differences in
frequency and differentiate significant ones from random noise.
It was already well established prior to
this study that income is partly heritable. What the authors wanted to do was
identify some of the causal genetic variants, see what other traits or life
outcomes might be mediating that causal connection, and illuminate the types of
genes and biological processes involved. They set out their causal model in
Figure 1 of their paper:
This figure shows that the idea that income
is partly genetically driven is not so preposterous after all. It follows
directly from the fact that intelligence is strongly heritable, that
intelligence is a contributing factor in educational attainment, and that
intelligence and educational attainment together contribute to what kind of job
someone gets and what they earn. None of these relationships is deterministic
or complete – they are all just partial correlations, but they’re real and
robust. When the causal pathway is shown like that, it would be amazing if
income were not partly heritable!
(Note: That doesn’t mean that that diagram
is necessarily correct or complete, however. It makes a lot of assumptions, one
of which we know is violated. More on that below).
The abstract sets out the findings of the
paper:
Socioeconomic position
(SEP) is a multi-dimensional construct reflecting (and influencing) multiple
socio-cultural, physical, and environmental factors. In a sample of 286,301
participants from UK Biobank, we identify 30 (29 previously unreported)
independent-loci associated with income. Using a method to meta-analyze data
from genetically-correlated traits, we identify an additional 120
income-associated loci. These loci show clear evidence of functionality, with
transcriptional differences identified across multiple cortical tissues, and links
to GABAergic and serotonergic neurotransmission. By combining our genome wide association
study on income with data from eQTL studies and chromatin interactions, 24 genes
are prioritized for follow up, 18 of which were previously associated with
intelligence. We identify intelligence as one of the likely causal,
partly-heritable phenotypes that might bridge the gap between molecular genetic
inheritance and phenotypic consequence in terms of income differences. These
results indicate that, in modern era Great Britain, genetic effects contribute
towards some of the observed socioeconomic inequalities.
So, what does all this mean? Let’s take a
look at the results themselves, the conclusions based on them, and the broader
implications.
The
results
The main general findings are that income
is partly heritable and that variation in a measure of intelligence (results on
a cognitive test) partly mediates those genetic effects. I think we knew both
of those things already, and they’re certainly not surprising, but the authors
may rightly claim that this is a more explicit demonstration than has been made
previously. Because they identify some associated variants they are able to use
somewhat different approaches (such as genetic correlations and Mendelian
randomisation) to try and tease out the causal relationships.
I’m a little skeptical of those kinds of
analyses in this context, or at least think the conclusions we can draw from
them are somewhat limited. The idea of genetic correlations is that when you find
some genetic variants associated with one phenotype (such as income) you can
then see whether those genetic variants are also associated with another
phenotype (such as intelligence) and measure how strongly the effects of the
variants correlate across the two phenotypes. In this case, the authors find a
strong genetic correlation (rg=0.69)
between intelligence and income, and comparable genetic correlations between
income and health and longevity.
That’s fine, but that number can be
misunderstood. It doesn’t imply that the actual overall correlation between intelligence and income is that strong.
It doesn’t imply anything about that correlation in fact. The genetic variants
collectively only “explain” a fraction of the variance in each of the two
phenotypes. That effect may well be highly correlated across the two
phenotypes, but still very minor and not sufficient to drive a strong overall
correlation between the two phenotypes.
You see a lot of these genetic correlations
in the literature these days, because they’re easy to do, especially in
databases with rich and diverse phenotyping, like the UK Biobank. The results
can definitely be interesting and informative, but it’s worthwhile keeping the
primary effect sizes in mind when interpreting them.
The authors also use a statistical
technique called Mendelian randomisation to try and extract a causal relationship
from what is otherwise just correlative data on genetic effects on intelligence
and income. The application of this technique here rests on some assumptions,
the main one being that variants that affect intelligence (which could thereby
affect income) do not also affect some other traits that could have independent
effects on income. The authors argue against this possibility of “horizontal pleiotropy” in their discussion
of limitations of the study, but it seems very difficult to rule it out. That’s
not to say there’s really any reason to doubt that model, as it is inherently
plausible, and a wealth of data shows that intelligence partially correlates
with future earnings.
Beyond those general points, the main
novelty of the paper lies in the identification of specific SNPs that are
associated with the phenotype of income. As mentioned, many of these are also
associated with intelligence, which is argued to be the causal mediator. When
you find an associated SNP (or multiple ones in a small region) you can often
deduce what gene is likely affected by those genetic variants. The authors
identify 144 genes in this way that harbour genetic variants statistically
associated with income.
What
kinds of genes are involved?
So, what kinds of genes are they? What are
their normal functions? Well, the first thing to note is that they are highly
enriched for genes expressed in the nervous system. That’s good news,
methodologically speaking, because it provides a crucial reality check. One
danger in performing GWAS for a social phenotype like income is that that
average income might vary geographically across the country. (In fact, we know
it does for the UK). This means you could pick up SNP associations that are
really tracking local differences in ancestry, rather than anything in a real
causal chain to the phenotype.
The authors use a number of statistical
methods to control for that problem but there’s always a worry that such measures are insufficient. However, if the GWAS signals were just
due to cryptic population stratification you would not expect enrichment for
any specific classes of genes – just signals all over the genome with no
biological meaning and possibly no biological effect at all. So, the enrichment
for neural genes strongly argues that the signals detected are real and also
fits with the favoured explanation that intelligence is a causal mediator.
Beyond that, however, there’s not really a
lot that can be said about these genes that is particularly illuminating. First, the
associated genes were not enriched for any particular biochemical functions. Second, the
authors use a variety of gene expression datasets to look for any enrichment of
associations in genes with higher expression in particular parts of the brain
or cell types. And they do come up with some statistically significant
enrichments, but, to be frank, as a neuroscientist, I don’t know what to make
of that information. I think probably nothing.
For example, the associated genes show
enrichment for “expression
differences in cerebellar hemisphere, at Brodmann area 9 (BA9) of the frontal
cortex, the nucleus accumbens and at Brodmann area 24 of the anterior cingulate
cortex (BA24)”. These findings rely on databases of gene expression
profiles across the brain. But most genes are expressed in many different areas
(many in all). Some are a bit higher in some areas than others, but that does
not mean their function is restricted to the areas with higher expression. Those
statistical descriptors do not reflect the true richness and dynamics of gene
expression in the brain. And the data presented in this paper are statistical
enrichments of those statistical enrichments (i.e., there’s a bit more signal
in genes that are expressed a bit more in those areas). It is highly doubtful
that the genetic effects on income (via intelligence or other psychological
traits) are mediated in any specific way (or even predominantly) by changes in
those highlighted brain areas.
The same can be said for the data on cell
type enrichments:
“Cell-type-specific analysis revealed that the expression that was
specific to the serotonergic neurons and to medium spiny neurons was associated
with income. Medium spiny neurons have previously been linked to schizophrenia,
which has a strong cognitive component and has previously been linked to
glutamatergic systems, including the N-methyl-D-aspartate receptor signalling
complex. Medium spiny neurons are a subtype of GABAergic inhibitory neurons.
Future work should examine if, like other cognitive traits, income is linked to
both GABAergic and glutamatergic systems.”
This is a strange way of working round to
the idea that maybe the two major classes of neurons in the brain are involved
somehow. In any case, the same problems apply as with the brain area expression
data – the real patterns of gene expression are simply not that specific. Cell
types are defined by combinatorial profiles of gene expression, and most
individual genes are expressed all over the place in many different cell types,
in complex and dynamic ways.
I don’t mean to be overly critical of the
attempts. These kinds of analyses might have thrown up some specific information
– they certainly have for other kinds of phenotypes. But for complex
psychological phenotypes, which really reflect the function or performance of
the whole brain, we should not expect any anatomical or cell-type specificity,
and my interpretation of the data presented in this paper is that, while there
are some statistically significant signals, they are not biologically
meaningful.
Finally, the authors integrate various
sources of data to focus on a subset of the genes that seem most likely to have
genetic variants directly affecting expression in the brain. They say: “These 24 genes therefore should be prioritized in
follow-up studies”. It’s not obvious what such follow-up studies might
entail. The effects of each of the variants on the phenotypes of interest are
individually almost negligible, and provide no real experimental purchase. Of course one can look at the effects of the genetic
variants on expression of the genes, and one can perform various studies to try
and figure out what the normal functions of those genes is, at a biochemical or
cellular or developmental level.
But that is a very different
question from asking what are the effects
of genetic variation in the gene. These effects can be very non-specific
and not really related to what you would say the function of the gene is. The genomic program of brain development involves lots of genes
with specific developmental functions but also relies on maybe ten-fold more
genes with much more generic functions – not really part of the instructions of
brain development per se, just general stuff required for everything to go
right (like metabolic enzymes, for example). Most of the genetic variants affecting brain development will thus
be doing so indirectly and non-specifically (and non-informatively).
So, I am not convinced that the
identification of these associated genes actually sheds much light on the
underlying biology driving the association with income (via intelligence or
otherwise), except to reinforce the idea that those kinds of psychological
traits are really emergent properties of the whole neural system and can’t
necessarily be tied to specific molecular systems.
The
conclusions
The final line of the abstract states: “These results indicate that, in modern era Great
Britain, genetic effects contribute towards some of the observed socioeconomic
inequalities.” This is clearly the intended take-home message. And it is true, in a literal sense, (if the main results are methodologically
sound, as they seem to be), but also a bit misleading and open to
misinterpretation. A more accurate statement would be that “genetic effects contribute
a minor amount towards some of the observed socioeconomic inequalities”.
Based on their calculations, the total
heritability of income that can be tagged by common genetic variants in the
population sample under study is only 7.4%. And using polygenic scores based on
the results of the GWAS to predict income in an independent sample only
explained 2% of the variance. Given that, the unqualified wording of the
abstract leaves open the possibility of people interpreting the effects as
larger than they really are. (And then running with that interpretation to make
all kinds of other claims).
Note that the overall genetic effect may in
fact be a good bit larger, as twin studies have estimated the total heritability
of income at 40-50%. These studies account for non-genetic familial effects, in
that these should be shared equally between twins regardless of whether they
are monozygotic or dizygotic. The observation is that pairs of MZ twins are
much more similar in income than DZ twins, implying a genetic effect. A likely
explanation for why the current study could not account for all of that
heritability is that much rarer genetic variants are responsible for much of it.
However, it is extremely important to
emphasise that the heritability is not a fixed number. There is no right
answer. It only measures the proportion of phenotypic variance that can be
attributed to genetic differences within
the population sample actually being studied. It cannot be generalised to
other populations or taken as a sweeping statement of truth. Variation in a
social measure like income can obviously be hugely dependent on social and
cultural factors – much more so in some settings than others. If you take a
very culturally homogeneous sample, where the variation in such social and
cultural factors is low, then the heritability of the trait will be
correspondingly higher (because it is a proportion). But across more diverse
social samples, the heritability could be much lower. And heritability within
one population says nothing – at all – about the origins of differences between
populations.
A concern is that people will take only the
widest, most sweeping interpretation of the results described here to bolster
the argument that socio-economic success is simply meritocratic, in the sense
that it is determined in some simple way by people’s innate intellectual
abilities and aptitudes. (See here for an example of such an argument). The reality is vastly more complex. Not only are environmental factors
hugely important, but they interact with genetic factors in complex, non-linear
ways.
This is where the authors’ basic model as
presented in Figure 1 is too simple, in my view. The models used to partition
variance assume that the effects of genetic and environmental factors will be
independent of each other and will simply add up. It’s difficult to determine
whether or not the assumption of additivity actually holds in a study like
this, but it seems likely that the effects of some
environmental factors will vary depending on a person’s genetic make-up, and
vice versa. But more importantly, there are correlations between a person’s
genes and the environment they experience. These arise because they share genes
with their parents, who partly create the environment for their children. I
think a more accurate causal pathway diagram would look like this:
The authors do say that they cannot rule
out such dynastic effects (gene-environment correlations across generations) as
a possible confounder and refer to another paper that suggests they may be
especially important in analyses of the genetics of educational attainment. The problem is the magnitude of such effects is extremely
difficult to calculate in the population study design employed.
This means the straightforward causal pathway – where
the genetics of the individual drives the heritability of income – is likely
too simple. The real scenario is sure to involve complex positive feedback
loops that entangle and amplify the effects of genetic endowments and social
capital over generations.
Finally, the authors add these important
caveats on how their results should be (and should not be) interpreted:
“A further limitation is that molecular genetic analyses of phenotypes,
such as intelligence, income or SEP, appear prone to being misinterpreted. Such
misunderstandings include describing associated variants as genes for income,
or the misinterpretation that any associated variant, and indeed any nonzero heritability
estimate, is evidence for genetic determinism or the immutable nature of these
phenotypes via environmental intervention. We include a figure (Fig. 1) that
illustrates that genetic variants do not act directly on income; instead,
genetic variants are associated with partly heritable traits (such as
intelligence, conscientiousness, health, etc.), which have their own complex
gene-to-phenotype paths (including neural variables) and are ultimately associated
with income. Therefore, the genetic variant–income associations discovered here
are no more for income than they are for these other traits. For more
discussion of the implications of these results, aimed at the general reader,
we have provided a Frequently Asked Questions (FAQ) document in Supplementary
Note 2.”
The FAQs are very useful, but it seems
unlikely that many readers will actually even notice they exist or go to the
trouble of digging them out, meaning that despite these efforts by the authors,
these findings are sure to be misinterpreted and misrepresented. I do think
some of the plainer language and important disclaimers in the FAQ answers could
have been included in the main text to better pre-empt misunderstandings.
I’m sure this paper will continue to
attract a lot of attention and we will see more studies like it (like this one,
for example) that explore the potentially fraught areas of genetic effects on
complex social outcomes.
Comments
Post a Comment